Speaker: Dr Damjan Vukcevic, University of Melbourne
Time & Date: 12:00pm Thursday 18 June 2020
Venue: zoom meeting, see details below
A common task in health and medicine is the classification of patient information into one of several categories by a trained expert. This could include assessing the presence and type of a tumour from a medical image or providing a disease diagnosis from a series of medical tests. Often such judgements are hard to make and error prone: two experts may rate the same scenario differently or the same expert may provide alternative ratings of the same scenario when rating it multiple times on different occasions.
Analysing the performance of such expert ‘raters’, and the accuracy of their ‘ratings’ across a series of ‘items’, is a common theme in much of the health and medical literature, especially in the setting where the true underlying category is unknown. Existing approaches, such as Cohen’s kappa, focus only on assessing inter-agreement, and have known problems stemming from the lack of any notion of underlying truth and the difficulty of coping with repeated ratings by the same rater.
Here we present and implement methods that explicitly model an underlying true category for each item and can cope naturally with any number of ratings for each item, including repeated ratings by the same rater. We implement Bayesian versions of these models using the probabilistic programming language Stan, and create an R package to fit and interrogate the output of these models.
Using real and simulated datasets, which are designed to mimic a wide range of medical scenarios, we test the performance of these models in estimating the true class of each item. We also explore situations such as having raters with much poorer accuracy, and comparisons with other (non-model-based) approaches.
========
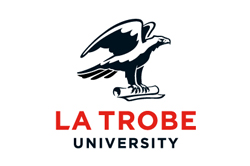
